- Home
- >
- Software Development
- >
- Machine Learning Poised to Impact Business Analytics in 2017 – InApps Technology 2022
Machine Learning Poised to Impact Business Analytics in 2017 – InApps Technology is an article under the topic Software Development Many of you are most interested in today !! Today, let’s InApps.net learn Machine Learning Poised to Impact Business Analytics in 2017 – InApps Technology in today’s post !
Read more about Machine Learning Poised to Impact Business Analytics in 2017 – InApps Technology at Wikipedia
You can find content about Machine Learning Poised to Impact Business Analytics in 2017 – InApps Technology from the Wikipedia website

Brian Wheeler
Brian Wheeler oversees the technology team at Morpheus Data. Prior to Morpheus, Brian founded a software development consulting firm which designed and developed solutions for a variety of industries including power grid management, ticketing systems, online trading, social networking and gaming, SOX compliance, and e-commerce. Brian holds a bachelor’s degree in Chemistry from Pomona College.
We may be years away from the “AI-enabled Coworker,” but the first implementations of machine-learning capabilities are finding their way into the everyday data-analysis tools used by businesses of all types. Cognitive assistance promises to reshape business processes, but only if app development and deployment tools are adapted to support machine learning.
While it has become fashionable to hype AI as the next game-changing technology promising to have an impact greater than either mobile or cloud, the reality is that machine learning will be a long time coming to everyday business analytics. As with any sea change, cognition is likely to sneak its way into applications and processes in drips and drops. It looks like 2017 could be the year many businesses get their first hands-on experience with cognitive-learning business apps.
For example, IBM’s Watson elicited plenty of “oohs” and “aahs” when it beat the Jeopardy champions, but the AI-based platform drew praise of another sort with the introduction of business solutions at the recent World of Watson event, as NewsFactor pointed out. Watson’s professional series applies cognitive learning to the analysis of large data sets; it works in tandem with enhancements to IBM’s DB2 for transactional processing in analytical databases.
IBM may have gotten a bit of a jump on the field of vendors racing to bring machine-learning capabilities to business processes, but the contest has just begun. The real winners are line managers, who stand to benefit the most from AI-enabled business applications.
Business Tackles Cognitive Implementation Challenges
The three cornerstones of cognitive technology are machine learning, natural-language processing (NLP), and speech recognition. In an article on Open Source For U, systems architect Sanghamitra Mitra writes that machine cognition is intended to imitate human reasoning to automate judgment-based components of business processes. The goal is to augment human activities to give people more time to focus on the really tough problems, like where to hold the holiday party.
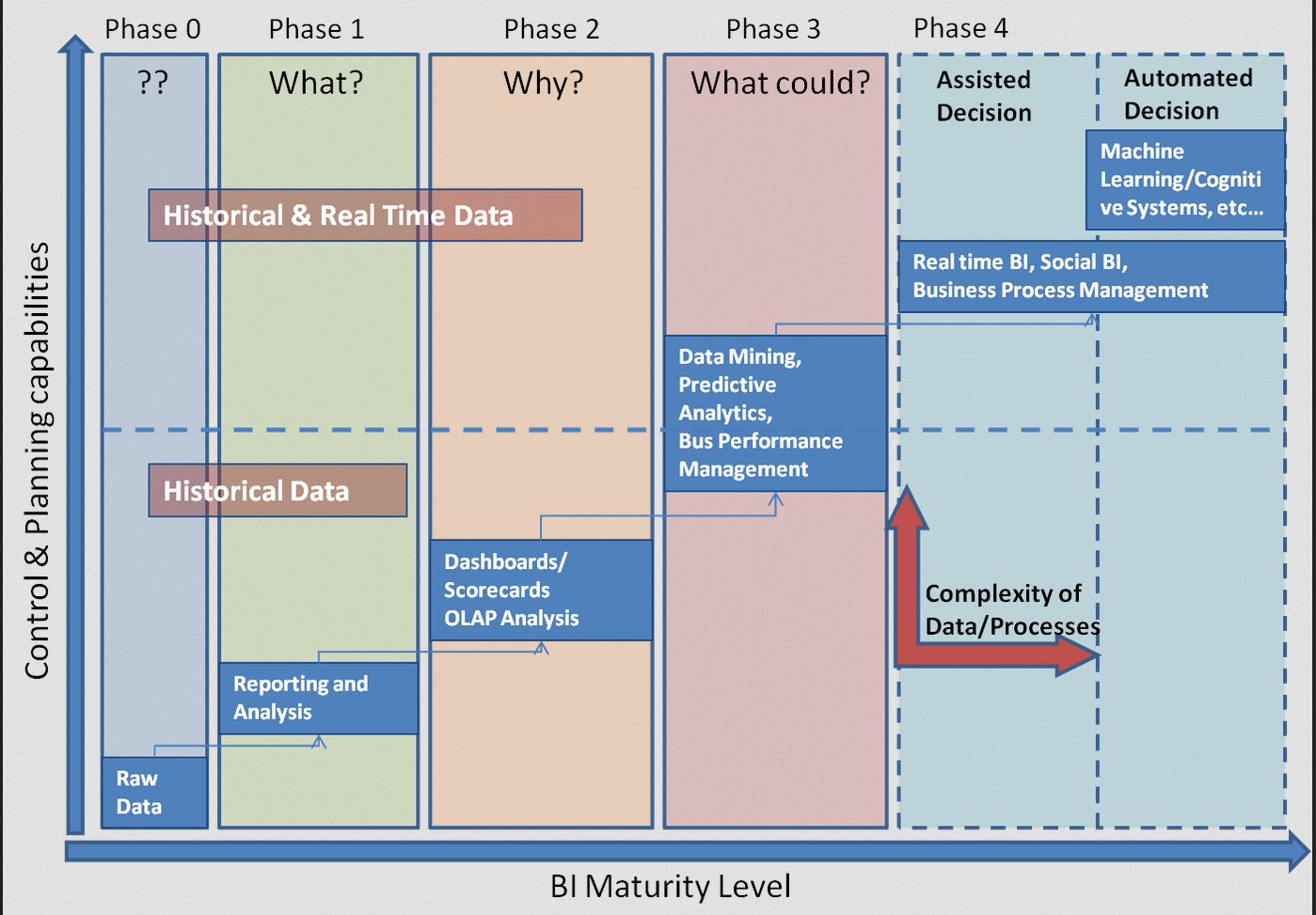
Machine learning extends the standard business intelligence maturity model to encompass real-time and social data, as well as business process management. Source: Jorge Garcia, via Wired Innovation Insights
The primary obstacle to implementation of cognitive systems is dealing with their inherent complexity. This fact is reflected in the cost of packaged machine-learning systems sold by vendors, as well as in the extensive infrastructure needed to support the systems. Several open-source alternatives have surfaced, providing enterprises with a quick, simple, and inexpensive way to dip their toe in the cognitive-computing water.
Here’s a quick look at popular open-source cognitive-learning tools:
- The R language and environment for statistical analysis is highly extensible and offers linear and non-linear regression, traditional statistics tests, time-series analysis, classification, clustering, and other statistical functions in addition to graphical features.
- Python is a high-level language that is popular with scientists and features machine learning implementations that fit well with the language’s agile and iterative approach.
- Apache Mahout serves as a useful environment for quick creation of scalable machine-learning applications.
- The H2O parallel-processing engine is used by data scientists and developers requiring fast, scalable machine-learning apps.
- The RapidMiner platform provides an end-to-end environment for implementing machine learning predictive-analytics models via a wizard interface.
Vendors Collaborate on AI Best Practices
There seems to be an inverse relationship between how much a new technology is hyped, and how well the technology is understood by would-be practitioners. In an attempt to remove some of the question marks surrounding machine learning and encourage adoption of the technology, Amazon, Facebook, Google, IBM, and Microsoft have joined to create the Partnership in AI program. The goals of the initiative are to support best practices, educate the public about AI’s potential benefits and costs, and “create an open platform for discussion and engagement.”
The best-practices proposals include ethics (fairness and inclusivity), transparency, interoperability, privacy, trustworthiness, reliability, and collaboration between people and AI-enabled systems. RedMonk analyst Rachel Stephens describes the AI research currently underway at several big-name tech firms:
- Facebook AI Research pledges to “contribute to the research community through publications, open source software, participation in technical conferences and workshops, and through collaborations with colleagues in academia.”
- Google’s AlphaGo neural network-based system beat the world’s best Go player earlier in 2016; the project is described in a Google-authored paper that appeared in the journal Nature in January 2016.
- Amazon has put up a $2.5 million prize for the development of an Alexa social bot that’s able to converse for 20 minutes.
- Microsoft recently created the AI and Research Group to be led by computer-vision researcher Harry Shum and intended to coordinate the research of more than 5,000 scientists and engineers working at the company.
- Salesforce is working on a project named Einstein that will integrate AI with CRM systems targeted at companies of all types and sizes.
Looking at the ‘Human Side’ of Machine Learning
Data scientists spend most of their time collecting and cleaning data, so early emphasis in machine-learning systems is on “simplifying and expediting” these tasks, as enterprise analytics consultant Thomas Dinsmore has pointed out. Unfortunately, existing data-warehousing workflows conflict with the data needs of machine-learning systems. This has led data scientists to create one-off, “just in time” extract-transform-load (ETL) workflows for individual projects.
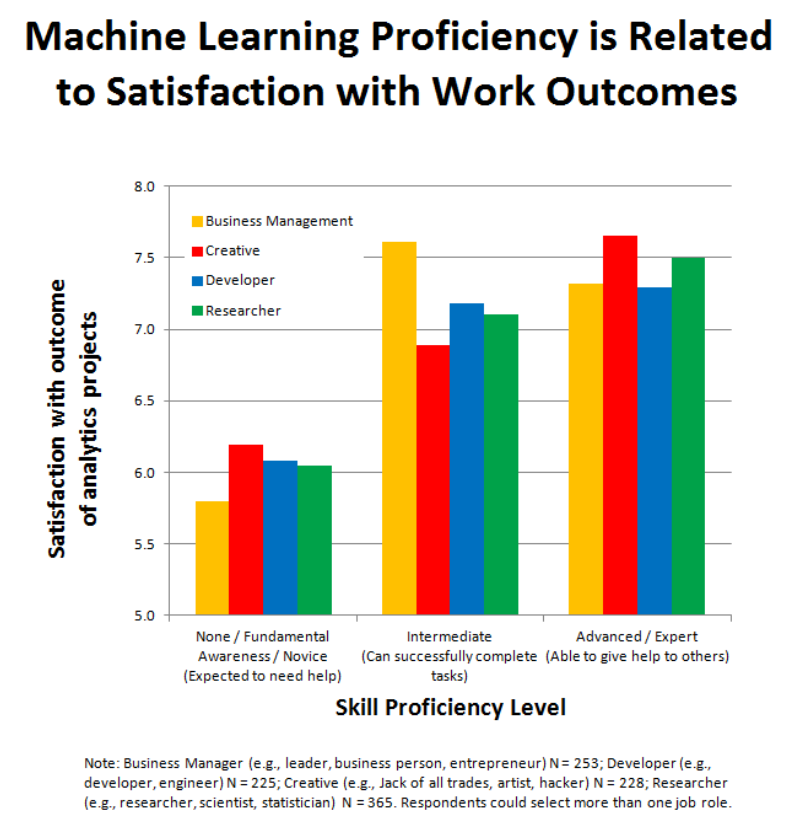
Proficiency with machine learning correlates to more positive outcomes across four categories of users: business management, creative, developer, and researcher. Source: Bob Hayes, Business Over Broadway
Machine-learning algorithms are not noted for their parallelism, so open-source tools such as Apache Spark require that the algorithms be rebuilt to distribute workloads across clustered servers. This is despite Apache Spark’s support for scalable data processing and ability to connect to a range of data platforms. Another obstacle to implementation of cognitive systems is the high level of processing power required for speech recognition, image categorization, and other deep-learning applications.
Yet the greatest hurdle still to be overcome has nothing to do with hardware or software: There simply aren’t enough data scientists to meet the demand. To address the shortage, the concept of the Citizen Data Scientist has been proposed, which would convert any business analyst into a data expert via the provision of graphical, “drag-and-drop” tools. Proponents of this approach include machine-learning vendors Alteryx, Angoss, RapidMiner, and Statistica.
Sophisticated Analytics Power in Managers’ Hands
The power of data management via a dashboard interface is shown by the Morpheus cloud application management platform, which lets you provision databases, apps, and app stack components on premises or in private, public, or hybrid clouds. Asynchronous provisioning allows multiple IT systems to be provisioned at the same time. Nodes can be added to databases and apps via the web interface, or using a command-line interface or API call; the databases and apps are reconfigured automatically as the nodes are added.
Of particular note to data analysts are the sophisticated logging, monitoring, and analysis tools built into Morpheus and accessible via the same intuitive interface. In addition to automatic uptime monitoring, application logs are collected automatically to facilitate introspection and troubleshooting. Last but not least, open REST APIs ensure smooth, seamless integration with heterogeneous systems.
IBM is a sponsor of InApps Technology.
Feature image by Joshua Earle via Unsplash.
Source: InApps.net
Let’s create the next big thing together!
Coming together is a beginning. Keeping together is progress. Working together is success.