The unprecedented rate at which enterprises are capturing information continues to outpace their capacity to analyze and use it. The proliferation of data types, sensors, operational devices, and applications that collect the data is fueling the status quo. This has greatly-amplified data integration challenges for digital businesses that require the modernization of their data integration strategy. They also require tools enabled by a metadata-driven approach to integrating data, regardless of its structure or source, in a hyperconnected infrastructure.
Why Metadata-Driven Integration Is Crucial
Metadata has become a critical component of modern data integration for several reasons including:
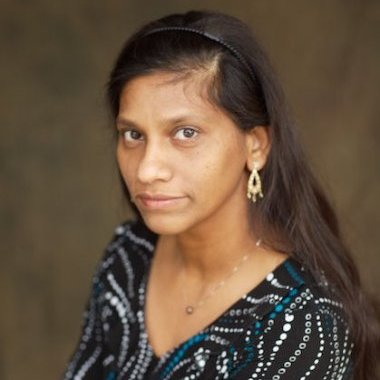
- The proliferation of devices and the distributed nature of data sources: has created an indispensable role for metadata. By supplying information pertaining to, among other things, (1) the devices that generate the data, and (2) the nature of the data being generated, metadata provides knowledge that is essential for data integration in a hyperconnected world.
- Diversifying integration strategies: are creating the need for multiple integration tools that must coexist and work together efficiently and effectively via metadata sharing (exchange) to achieve an enterprise’s data integration goals. Metadata enables cooperation among diverse, more-or-less specialized tools by providing visibility into both upstream and downstream processes.
- Exploiting data quality and governance processes as part of data integration: embedding these processes in the integration pipeline necessitates bidirectional sharing of metadata between the integration tools, and quality and governance tools.
- Performance optimization in integration scenarios: Metadata is providing knowledge of the characteristics of underlying sources in support of dynamic optimization strategies.
- Integration solutions incorporating logical data architectures: such as logical data warehouse. Leveraging metadata, enterprises can build multiple semantics relevant to diverse business units in support of BI and analytics.
Machine Learning Drives Automation in Integration
Although machine learning presently occupies only a minor role in data integration, it is poised to accelerate in importance given its potential to drive automation within the framework of the modern data integration paradigm. Currently, there are two ways that enterprises are leveraging machine learning in data integration:
- Embedding machine learning components in integration flows or pipelines to support real-time analytics and decision making.
- Leveraging machine learning to minimally automate the integration components including automatic:
- Data cataloging and data characterization (e.g., inferring schemas and structure)
- Transformation recommendations
- Metadata mapping
Metadata and Machine Learning Together
The future of data integration lies in greater automation with a goal of increasing end-user productivity and reducing the time expended on building integration workflows, resulting in overall reduction of integration costs and improved agility.
As depicted in Figure 1, automation of data integration at present is minimal, exemplified by generating and cataloging metadata to establish data lineage, inferring schemas and relationships, and suggesting transformations. In the near future, automation will grow to encompass discovering and automating of data quality and governance rules, and data transformations, but will still represent only partial automation of data integration. Thereafter, the extent of further automation will depend on vendors’ commitments to investing in modernizing their products.

Figure 1. The progression of automation in data integration (DI) fueled by metadata and machine learning.
Metadata and machine learning work together to drive data integration automation. Metadata provides crucial information and insights, such as characteristics of the data, such as format, location, relationships, data quality, and usage information. Machine learning leverages this information to make and automate recommendations pertaining to integration tasks. When used together, organizations can exploit the benefits of each, enabling them to capture and apply information to their competitive advantage.
Feature image by Pietro Jeng via Unsplash.